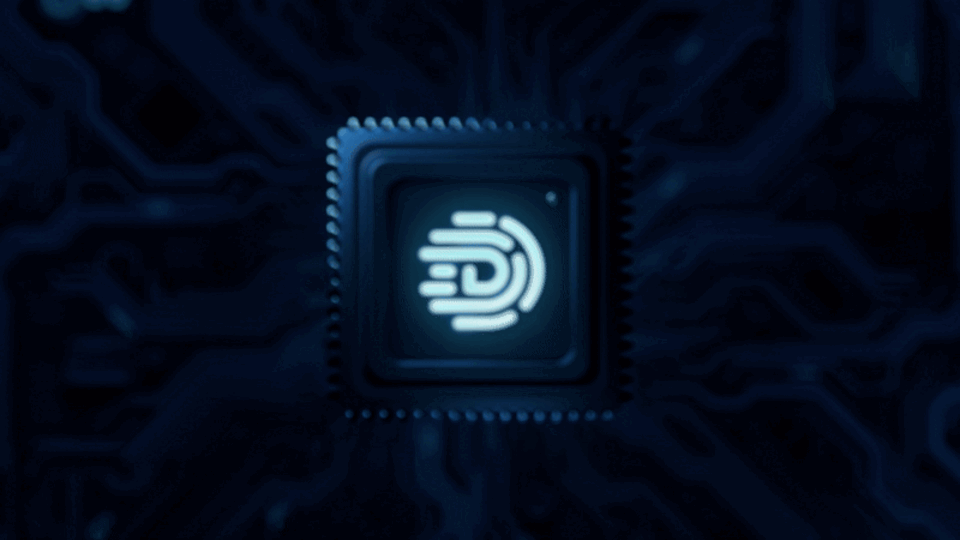
GPT-4o vs. Digits AI: The Power of $700 Billion worth of transactions
At Digits, we're on a mission to automate accounting—the tedious parts that nobody enjoys, such as transaction categorization, bank reconciliation, and yes, even paying bills. We deliver live dashboards and insights, intuitive financial reports, and our 24/7 AI finance advisor to thousands of startups and small businesses, including over 1,500 accounting firms and their clients.
Today, we're excited to share a major growth milestone: Digits has now processed over $700,000,000,000.00 worth of financial activity (yes, that's 700 Billion dollars!) across over 5,000 startups and small businesses—representing over 135 Million unique, double-entry transactions.
We've been overjoyed by the support we've received on this journey, not just from the tech and VC/investor communities, but from the broader accounting industry as well, up to and including the AICPA. In June, the Digits team will present our work at AICPA Engage as well as give the keynote address at Scaling New Heights. We look forward to seeing you there!
Automating Accounting 🧮
Today, we're excited to share publicly, for the first time, how we approach automating accounting and how Digits AI competes with the world's leading Large Language Models (LLMs).
While ChatGPT has captured the world's attention over the past 18 months, and opened eyes to the power of "AI" (aka machine learning), the Digits team has been heads-down developing AI Bookkeeping technology since 2018.
Back in 2019, we were awarded a patent for self-improving regular expression models—an early incarnation of machine learning algorithms that is now (thankfully!) obsolete. The next year, in 2020, we began training in-house deep-learning models (what most people now refer to as "AI"), and our ML Engineering team now trains, deploys, and maintains a full suite of proprietary models and autonomous agents, which work in concert to automate large portions of the bookkeeping and monthly close process.
Benchmark Showdown 🥊
With the recent release of GPT-4o, we took the opportunity to conduct extensive benchmarking tests to evaluate industry-leading LLMs like OpenAI's GPT-4o & GPT-4 Turbo and Meta’s Llama3 on common bookkeeping tasks.
For these tests, we developed a list of 1,000 transactions based on real data, encompassing a diverse and representative set of startup and small business financial activity. The AI models were tasked with correctly categorizing the transactions against a standard chart of accounts while we measured their speed, accuracy, and hallucination rate. The outcomes were then compared against results provided and verified by professional accountants following US GAAP standards.
Here's how the models stacked up:
Correctly Categorized Transactions:
- GPT-4 Turbo: 61.7%
- GPT-4o: 59.9%
- Llama3: 42.1%
Correct category in 3 top suggestions:
- GPT4-Turbo: 78.20%
- GPT4o: 69.50%
- Llama3: 61.20%
Made-up ("hallucinated") Categories:
- GPT-4o: 0.1%
- GPT-4 Turbo: 1.6%
- Llama3: 7.87%
Speed:
- GPT4o: 1.39 seconds/prediction
- GPT4-Turbo: 4.02 seconds/prediction
- Llama3: 7.10 seconds/prediction
And the winner is… 🏆
Overall, these models performed decently. While GPT-4 Turbo was slightly more accurate at overall categorization, GPT-4o is notable for how dramatically faster and less prone to hallucination it is. We're excited to see OpenAI, Meta, and Anthropic continue to push the bounds on speed, accuracy, and hallucination rate in future models and we plan to update these benchmarks as new models are released.
When it comes to accounting, however, decent is just not good enough. You would quickly fire any accountant that miscategorized 38% of your transactions! So, how do we do even better?
Introducing Digits AI Bookkeeping 🧮
Large Language Models are truly remarkable technologies, capable of a vast range of tasks—but that is also their Achilles' heel; they are generalists by nature, and can struggle when applied to specific, structured fields like accounting, as seen in the benchmarks above.
When it comes to bookkeeping, trust is paramount: you need to know that your books are clean and accurate, period. Any technology that fails to deliver trust is effectively worthless to the industry, so there is a high bar to introducing new tools into the monthly close process.
At Digits, we have pioneered purpose-built AI Bookkeeping models, trained and evaluated purely on double-entry accounting tasks. These are fundamentally different from generic Large Language Models, and are designed with the following goals in mind:
Custom to each business:
Every business is different, so booking against a standard chart of accounts is not that useful in the real world. AI Bookkeeping models must quickly learn the intricacies of every business, and perform well against arbitrary, complex, and custom charts of accounts.
Highly repeatable output:
Once the AI learns how to categorize a transaction, it needs to do so repeatedly and reliably, every month going forward, until taught otherwise.
High confidence or bust:
Mistakes are unacceptable, because at scale, they are very difficult to catch. We would rather the AI give up (and drop the transaction into a human review queue) than have it make a guess and get it wrong.
No hallucinations:
Fabricating category or vendor names is the fastest way to destroy trust, so we've developed a system architecture to detect and prevent hallucinated output.
Security at every level:
We've pioneered techniques to train and validate our models against encrypted data, and we go to extreme lengths to minimize data disclosed to any 3rd-party, including OpenAI.
No single model excels across all of these dimensions. Instead, we train and fine-tune purpose-built models (such as NER transformer models and similarity models) for a range of tasks, and orchestrate them together to take advantage of each of their strengths.
The result of this systems-based approach is dramatically improved performance—Digits AI correctly categorizes 91% of transactions with a 0% hallucination rate, and we are actively working on efforts to push its accuracy even higher in the coming months.
No AI will ever be perfect, and human accountants will always play a critical role in the monthly close process, so we do not expect this to ever reach 100%. The real world is simply too messy, and every business, no matter how small, is unique. But if we can automate 90%+ of the tedium, whether it's transaction categorization, bank reconciliation, or payables and receivables processing, that will dramatically improve the day-to-day lives of millions of accounting professionals around the world and save startup founders and small business owners countless time and money.
Collaborations and Industry Engagement 🤝
Our advancements in AI Bookkeeping would not be possible without strategic industry partners, and we are committed to ongoing collaboration to push the state of the art forward:
NVIDIA Partnership:
Our partnership with NVIDIA, highlighted at their booth during Google Next '24, showcased how integrating their cutting-edge GPU technology with our machine-learning models enhances accuracy and efficiency. This collaboration is instrumental in driving AI advancements, especially in complex fields such as double-entry accounting.
Google I/O Contributions:
As Google AI Advisory Board members, we had the privilege to engage directly with the AI/ML community at Google I/O '24. We exchanged insights with the Developer X group and explored significant advancements like the Gemma 2 LLM, Responsible Generative AI Toolkit, and the LLM Comparator tool.
Experience AI Bookkeeping 👀
If you're excited about the promise of accounting automation, and want to experience the power of AI Bookkeeping first-hand, we would love to talk!
We work directly with US-based, VC-backed tech startups to fully automate their accounting:
And we partner with top accounting firms to bring modern accounting automation to the full breadth of US SMBs: